Research
Multiscale imaging of organ-specific inflammation
Examples
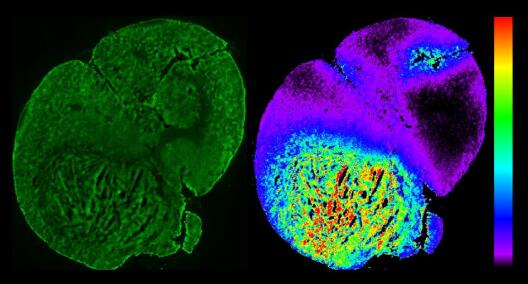
Blood vessels from the brain tissue of a mouse. This visualisation is based on images which were taken using lightsheet microscopy and show layers of a tissue sample (here a section of about 100 layers on 550 x 550 x 300 µm). To contrast the blood vessels, an adhesion molecule in their endothelial cells was stained. We merged the microscopy data into a 3D image on the computer and used the hysteresis thresholding method to segment the blood vessels, i.e. to digitally delineate them from other tissue regions. The image serves as a reference for new image processing methods utilising machine learning to improve segmentation. (Project B04)© Daniel Beckmann/Benjamin Risse's research group, microscopy data by Nadine Bauer/Friedemann Kiefer's research group Immune cells (neutrophils) infected by enteric bacteria (Yersinia pseudotuberculosis) in a cell culture. Confocal microscopy shows the neutrophil’s cell nuclei (blue), actin (the “skeleton” of the cells, orange) and the bacteria (green). These bacteria lack a specific gene, allowing the immune cells to take them up more efficiently. We are investigating, for example, whether such bacterial mutations give neutrophils an advantage in eradicating the bacteria from the body or whether they help the bacteria survive these immune cells’ attacks. (Project C07)© Héctor Álvarez Manzo / Petra Dersch's research group Blood vessels in the kidney tissue of a mouse. This abstract visualisation is based on images of a tissue sample taken using lightsheet fluorescence microscopy and was generated using 3D image processing techniques. These techniques were developed as part of our project to handle large high-resolution datasets in a way that enabels a simultaneously coarse and fine-scale analysis of vessel structures and quantitative comparison between multiple datasets. Here, the vessel tree volume was segmented before extracting the topology and specific vessel properties. The light blue spheres mark the ends and branch points of the blood vessels. A colour scale visualizes the different diameters of the vessels. (Project Z01)© Dominik Drees/Xiaoyi Jiang's research group, microscopy data by Nils Kirschnick/Friedemann Kiefer's research group Imaging of inflammation using microscopy (left, immune cells clustering in the belly of a mouse) and whole-body imaging (PET-CT, right, accumulation of radioactively labelled sugar around a patient's blood vessel prosthesis). Using microscopy, only a spatial snapshot of the organism can be analysed, using whole-body imaging individual cells cannot be distinguished. We aim to develop methods that allow for the longitudinal analysis of large tissues and whole organs with cellular resolution and for the compiling of information provided by different imaging modalities.© CRC inSight / Friedemann Kiefer's and Michael Schäfers's research groups
Whenever tissue is damaged in the body – e.g. through infarctions, autoimmune diseases and infections – inflammation occurs. It is the immune system’s instantanious reaction to external stimuli and acute dangers and, in principle, something positive: inflammation limits damage and promotes the healing process. But the body is not always able to bring the situation under control and inflammation can become life-threatening or chronic, permanently impairing the function of organs. A challenge medicine is facing, at present, is to predict how inflammation will progress.
We are analysing when and where different immune cells become active in the organism when inflammation occurs, via which molecular mechanisms they interact with each other and their environment, and which specific functions they have in the inflammation of different organs. In tackling these questions, we incorporate different imaging technologies – both microscopic and whole-body methods – into our investigations and integrate information gathered from the individual cellular level up to the level of the entire organism. We expect that this holistic view will allow us to identify links between cellular inflammation mechanisms and the function of organs. This “multiscale imaging” requires new chemical-biological strategies to label the same cell type, or even the same cell, with different signal transmitters, e.g. with fluorescent, magnetic or radioactive molecules, because these generate signals that become visible through different imaging technologies. New challenges are also posed by the need to integrate data sets from different imaging techniques to recognise complex patterns in cell behaviour on a holistic level. Mathematical models and the training of artificial intelligence – so-called deep learning – play an essential role here.
In the long term, we expect our results to lead to new diagnostic approaches for clinical imaging modalities such as nuclear imaging, magnetic resonance imaging and photoacoustic imaging. Such methods could help detect inflammation associated with, for example, heart attacks, autoimmune diseases that lead to inflammation of the joints, skin or intestines, and bacterial infections in the lungs or kidneys. They could finally help to find and assess the efficacy of an immunoregulatory therapy that is tailored to individual patients.