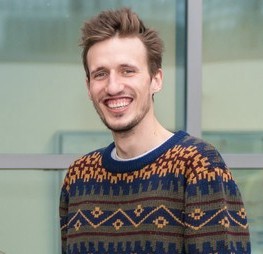
PhD Student
Computational Drug Discovery
M.Sc. Applied Statistics
E-Mail: janosch.menke@uni-muenster.de
Institute of Pharmaceutical and Medicinal Chemistry
Corrensstr. 48
48149 Münster
Research Topic
In my work, I focus on the improvements of molecular fingerprints using neural networks.
Molecular fingerprints are used in similarity search to quickly screen through databases in the hopes of identifying novel compounds with activity to a specific protein target. Traditional fingerprints lack the ability to encode domain-specific and/or target information, which could improve the results of the similarity search. My goal is to use neural networks to enrich traditional fingerprints with such domain-specific information and create novel fingerprints using Graph Neural Networks.
Publications
Menke, J., Homberg, S. & Koch, O. Introduction to artificial intelligence and deep learning using interactive electronic programming notebooks. Arch. Pharm. 2023, e2200628. https://doi.org/10.1002/ardp.202200628
Menke, J., Massa, J., & Koch, O. Natural Product Scores and Fingerprints Extracted from Artificial Neural Networks. Computational and Structural Biotechnology Journal. 2021, in press. https://doi.org/10.1016/j.csbj.2021.07.032
Menke, J., Maskri, S., & Koch, O. Computational Ion Channel Research: from the Application of Artificial Intelligence to Molecular Dynamics Simulations. Cell Physiol Biochem. 2021 55(S3), 14-45. https://doi.org/10.33594/000000336
Menke, J., & Koch, O. Using Domain-Specific Fingerprints Generated Through Neural Networks to Enhance Ligand-Based Virtual Screening. Journal of Chemical Information and Modeling. 2021, 61(2), 664-675. https://doi.org/10.1021/acs.jcim.0c01208
Mulder, J., Gu, X., Olsson-Collentine, A., Tomarken, A., Böing-Messing, F., Hoijtink, H., ... & van Lissa, C. BFpack: Flexible Bayes factor testing of scientific theories in R. 2019, arXiv preprint arXiv: 1911.07728.