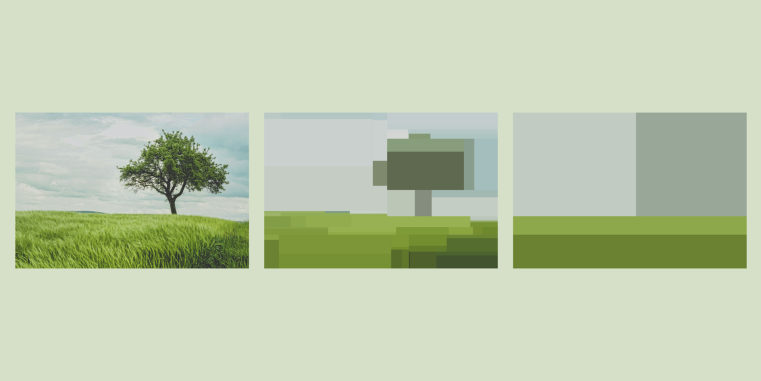
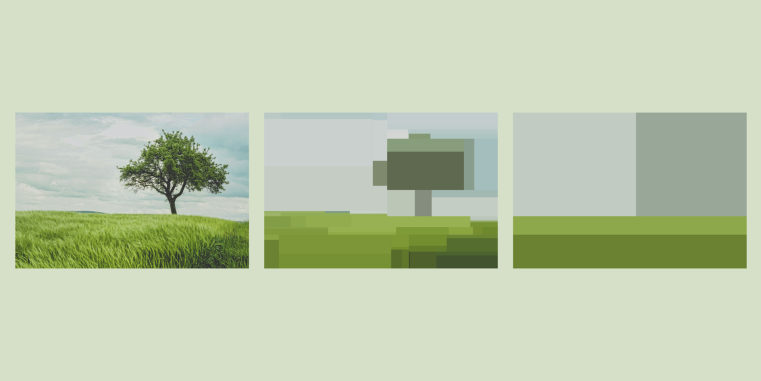
AI and Sustainability
The energy requirements of digital infrastructure are rising continuously; currently, the digital infrastructure worldwide emits twice as much CO2 as civil aviation. The increasing use of AI reinforces this trend, as the training of algorithms in particular is very energy-intensive. At the same time, AI algorithms can make significant contributions to the efficiency of energy use, or be used in data collection to analyze environmental changes. Therefore, from a sustainability perspective, a differentiated view on AI is necessary.
The perspectives on AI & sustainability can be divided into two approaches. On the one hand, perspectives that focus on the potentials of the technology for a transformation towards more sustainability (AI for sustainability) and, on the other hand, perspectives that deal with the (also unintended) impacts of AI applications on sustainability (Sustainability of AI) (cf. van Wynsberghe 2021).
Research on the potentials of AI for sustainability shows a wide range of possibilities in which algorithms can be applied, for example to improve agricultural yields, for reductions in resource consumption such as water or energy, and for organizing transportation. The networking of systems, for example in so-called smart cities, is also a possible application in which AI systems can contribute to increase sustainability. Another strand of research in this field is concerned with research on climate change and the prediction of changes caused by climate change.
Research on the sustainability of AI systems focuses on the impact of the technology on the environment and people. An important starting point is the high energy consumption of machine learning. As Strubell et al. (2019) showed, the computing power used to train NLP systems, for example, generates relevant CO2e emissions. Thus, the training of the NAS system generated about 284t CO2e. In addition to these emissions, there are also effects on the people in the mining areas in the supply chains of the required hardware and the necessary equipment for the AI systems. The analysis of the sustainability of AI systems also includes possible negative effects on certain groups in society. In this context, the analysis of the adoption of discriminatory patterns from training data plays a central role.
- van Wynsberghe, A. (2021): Sustainable AI: AI for sustainability and the sustainability of AI. AI Ethics 1, 213–218.
- Strubell, E.; Ganesh, A.; McCallum, A. (2019): Energy and Policy Considerations for Deep Learning in NLP. In Proceedings of the 57th Annual Meeting of the Association for Computational Linguistics, pages 3645–3650, Florence, Italy. Association for Computational Linguistics.
Courses
The courses on AI & Sustainability aim to shed light on the role that AI systems can play in a transformation towards sustainability by means of research literature and concrete examples.
The central questions are :
- How can AI applications contribute to the transformation towards sustainability?
- What trade-offs can arise between the development and application of AI systems and a more sustainable society?
- What constitutes "sustainable" AI?
- How can societal framework conditions be shaped in order to use AI applications sustainably?
Through exploring the potentials, but also possible trade-offs, students will develop a detailed understanding of the interrelationships. The seminars offered on this topic will be made accessible to students from different disciplines through the General Studies.
The courses will be held in the winter semester 2023 and summer semester 2024 and can be attended without prior knowledge. The teaching materials will be made available on Learnweb.
Blog post
As part of the project, Benedikt Lennartz and Shari Langner wrote a blog post on "Why a sustainability perspective on artificial intelligence is necessary".