Next: Analytical solution of mixture
Up: Prior mixtures for regression
Previous: High and low temperature
  Contents
Especially interesting is the case
of
-independent
=
and
-independent
.
In that case the often difficult to obtain
determinants of
do not have to be calculated.
For
-independent inverse covariances
the high temperature solution
is
according to
Eqs.(555,561)
a linear combination
of the (potential) low temperature solutions
 |
(566) |
It is worth to emphasize that, as the solution
is not a mixture of the component templates
but of component solutions
,
even poor choices for the template functions
can lead to good solutions, if enough data are available.
That is indeed the reason why the most common choice
for a Gaussian prior can be successful.
Eqs.(565) simplifies to
 |
(567) |
where
 |
(568) |
and (for
-independent
)
 |
(569) |
introducing
vector
with components
,
matrices
 |
(570) |
and constants
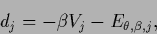 |
(571) |
with
given in (564).
Eq. (567) is still a nonlinear equation
for
, it shows however that the solutions
must be convex combinations of the
-independent
.
Thus, it is sufficient to solve
Eq. (569) for
mixture coefficients
instead of Eq. (548) for the function
.
The high temperature relation
Eq. (553) becomes
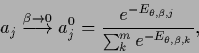 |
(572) |
or
for a hyperprior
uniform with respect to
.
The low temperature relation Eq. (559)
remains unchanged.
For
Eq. (567) becomes
 |
(573) |
with
=
in case
is uniform in
so that
=
, and
because
the matrices
are in this case zero except
.
The stationarity Eq. (569) can be solved
graphically (see Figs.7, 8),
the solution being given by the point
where
,
or, alternatively,
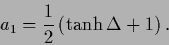 |
(575) |
That equation is analogous to
the celebrated mean field equation of the
ferromagnet.
We conclude that in the case of equal component covariances,
in addition to the linear low-temperature equations,
only a
-dimensional nonlinear equation has to be solved
to determine the
`mixing coefficients'
.
Figure:
The solution of stationary equation
Eq. (569) is given by the point where
=
(upper row) or, equivalently,
=
(lower row).
Shown are, from left to right, a situation
at high temperature and one stable solution (
=
),
at a temperature (
=
) near the bifurcation,
and at low temperature with
two stable and one unstable solutions
=
.
The values of
=
,
and
used for the plots
correspond for example to the one-dimensional
model of Fig.9 with
,
,
.
Notice, however, that the shown relation is valid
for
at arbitrary dimension.
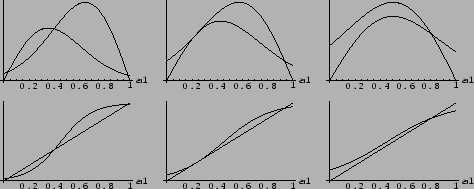 |
Figure 8:
As in Fig.7
the plots of
and
are shown
within the inverse temperature range
.
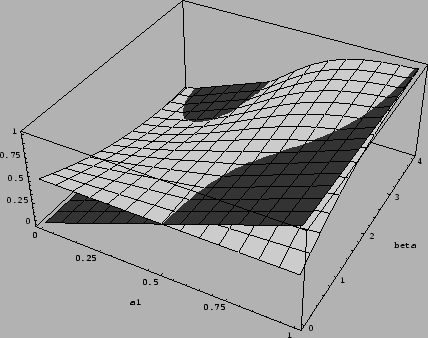 |
Figure 9:
Shown is the joint posterior density of
and
, i.e.,
for a zero-dimensional example
of a Gaussian prior mixture model
with training data
and prior data
and inverse temperature
.
L.h.s.:
For uniform prior (middle)
with
joint posterior
the maximum appears at finite
.
(Here no factor
appears in front of
because normalization constants for prior and likelihood term have
to be included.)
R.h.s.:
For compensating hyperprior
with
the maximum is at
=
.
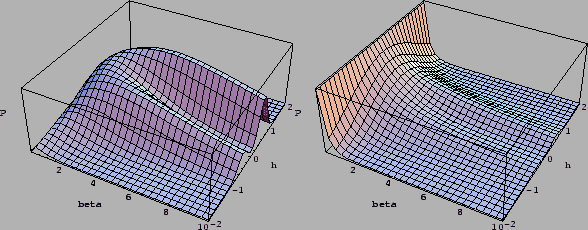 |
Figure 10:
Same zero-dimensional prior mixture model
for uniform hyperprior on
as in Fig.9,
but for varying data
(left),
(right).
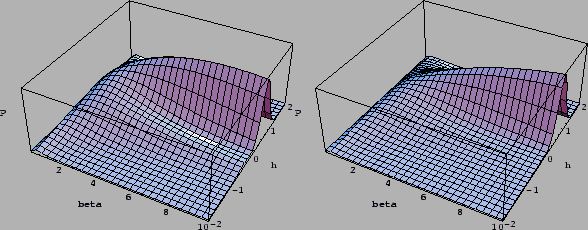 |
Next: Analytical solution of mixture
Up: Prior mixtures for regression
Previous: High and low temperature
  Contents
Joerg_Lemm
2001-01-21