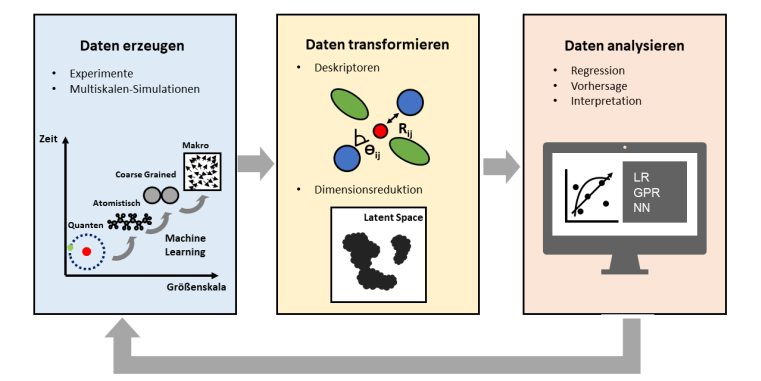
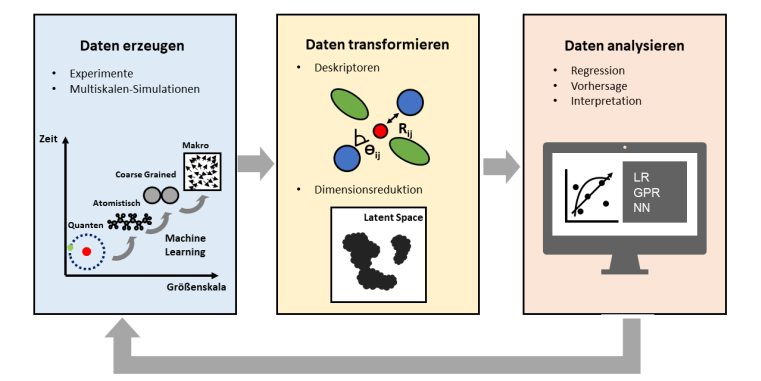
ML in the Theoretical Analysis of Molecular Dynamics
In recent years, the use of machine learning (ML) methods has become increasingly important in the field of (theoretical) analysis of molecular systems. In this context, ML supports both the data evaluation of experiments and the data generation using various simulation methods from the quantum chemical to the macroscopic scale. In the field of density functional theory and molecular dynamics simulations, ML models already outperform classical methods in terms of accuracy and simulation time. At the same time, ML models do not always supersede classical methods, but rather extend them and provide new approaches.
In the field of data evaluation, cluster algorithms and regression methods enable the recognition of highly complex correlations in high-dimensional spaces. Image recognition software can simplify or take over the evaluation of spectra. Ultimately, highly automated processes can be created that require minimal human intervention, enabling high-throughput experiments in which a computer uses Active Learning to decide which experiments to perform next.
In the coming years, we can expect to see an increasing number of applications and further exploration of the possibilities of machine learning in the field of molecular systems analysis.
Courses
In the module "Machine Learning in the Theoretical Analysis of Molecular Systems", various methods of machine learning are taught in a series of lectures held in the winter semester as well as in an associated practical course using selected examples. The lecture is intended for beginners in the field of machine learning who have a basic interest in theory (previous knowledge e.g. from the master modules "Theoretical Chemistry", "Quantum Theory of Solids" or "Elementary Excitations in Solids" is helpful but not mandatory).
The lecture is divided into several sections, each dealing with current methods and research questions in different subfields. One part of the lecture deals with how machine learning can help improve exchange-correlation functionals in the area of density functional theory. Another part deals with Machine Learning Potentials (MLP), which can be used to predict various quantum chemical and macroscopic properties of a molecule, providing an alternative to classical force fields, and can be used to derive coarse-grained potentials from atomistic potentials.
When analyzing data and trajectories, cluster analysis and dimension reduction can provide new insights into complex relationships. Using an example for characterizing structure-dynamics relationships, different algorithms are presented and compared. In addition, Active Learning is presented as a method to optimize system properties and automate the generation of new data.
The topics addressed in the lecture will be deepened in an accompanying practical course, where the different methods can be explored by the students themselves.
The lecture and the practical course take are face-to-face events. The course content (slides, scripts and Jupyter notebooks) can, however, be studied online at any time. Participation in lecture and practical course is required to receive credit points.
The course will be held for the first time in the winter semester 2023/24.