Next: Equal covariances
Up: Prior mixtures
Previous: Analytical solution
  Contents
Low and high temperature limits
are extremely useful because in both cases
the stationarity Eq.(21) becomes linear,
corresponding thus to
classical quadratic regularisation approaches.
In the high temperature limit
the exponential factors
become
-independent
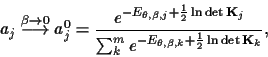 |
(34) |
(for
replace
by
).
The solution
is a (generalised) `complete template average'
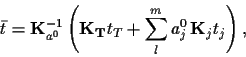 |
(35) |
with
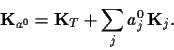 |
(36) |
This high temperature solution
corresponds to the minimum of the quadratic
functional
,
In the low temperature limit
only the maximal component contributes,
i.e.,
 |
(37) |
(for
replace
by
)
assuming
=
+
or
=
+
+
.
Hence,
low temperature solutions
,
are
all (generalised) `component averages'
provided they
fulfil the stability condition
 |
(38) |
or, after
performing a (generalised) `bias-variance' decomposition,
,
with
matrices
 |
(39) |
and (generalised) `template variances'
That means
single component averages
(which minimise
and thus
)
become solutions at zero temperature
in case their (generalised) variance
measuring the discrepancy between data and prior term is small
enough.
Next: Equal covariances
Up: Prior mixtures
Previous: Analytical solution
  Contents
Joerg_Lemm
1999-12-21