Probabilistic Modeling of Long-term Peatland Carbon Dynamics
DFG KN 929/23-1
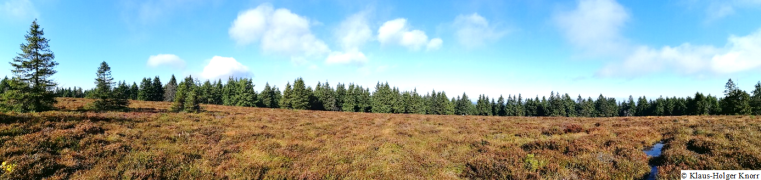
Peatlands regulate atmospheric greenhouse gas concentrations and thus the global climate. They form one of the largest terrestrial C stores and current and projected long-term shifts in temperature, precipitation, and nitrogen deposition represent a potential threat to these functions.
Dynamic peatland models (DPM) are needed to acquire a mechanistic understanding of process interactions, to predict long-term changes in C accumulation rates, and to synthesize contrasting results of individual studies. For about 40 years, DPM have continuously been improved by including additional processes, temporal dynamics, and spatial heterogeneity.
Sensitivity analyses of DPM have revealed that uncertainties are generally large yet crucial for a correct interpretation of process interactions. In other disciplines, the application of probabilistic models, uncertainty analysis, and uncertainty reduction via data assimilation has proven useful extensions of former deterministic models. However, uncertainty has barely been quantified and analyzed for probabilistic DPM (Fig. 1).
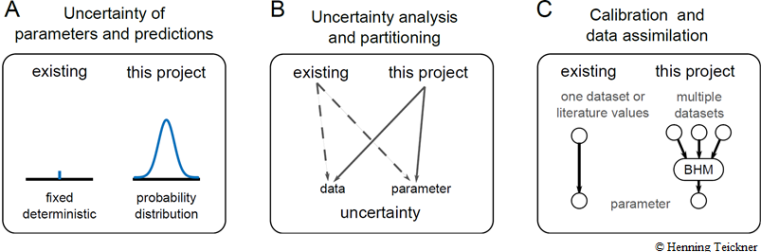
To make DPM more useful, we suggest to develop a probabilistic DPM (Fig. 1) and to quantify, analyze, and reduce uncertainties in its input data and parameters, using uncertainty analysis and data assimilation. We expect that data assimilation can reduce uncertainties especially for long-term decomposition rates if one synthesizes different existing data sources (peat core data and litter bag data) and the information provided by multiple peat properties at the same time (e.g. C and N content).
With this framework, we aim to assess the impact uncertainties have for our understanding of the effects of temperature, precipitation, and nitrogen deposition on peatland C accumulation, identify conditions under which experiments yield contrasting results, and provide strategies for efficient future uncertainty reduction in DPM.
Main research goals
- Development of data compendia to enable data assimilation for DPM and computation of mid infrared spectra (MIRS)-based calibration models.
- Development and application of MIRS-based calibration models to predict peat properties (e.g. element contents).
- Implementation of a probabilistic DPM (PDPM) for the prediction of long-term (decades to millennia) northern peatland C dynamics as BHM that reflects the structure of state-of-the-art DPM and considers uncertainty in the data, parameters, structure and code evaluation.
- Evaluation if data assimilation reduces uncertainties in estimated long-term decomposition and C accumulation rates if (a) litter bag experiments and peat core data are synthesized, and (b) in addition multiple peat properties are integrated into the model, in comparison to using only litter bag data.
- Analysis of the impacts of uncertainty in data and parameters (for different uncertainty sources) on the uncertainty in predicted C accumulation.
- Identification of conditions under which uncertainties do not support an unambiguous interpretation of the net effect of temperature, precipitation and N deposition on C accumulation rates. (b) Identification of conditions under which these factors can result in non-monotonic effects on C accumulation rates.
Main methods
- Probabilistic modeling with Bayesian hierarchical models.
- Uncertainty quantification and analysis, sensitivity analysis.
- Mid infrared spectra-based models to predict peat properties.
- Development of data compendia.
Project partners
Institute for Landscape Ecology, Ecohydrology and Biogeochemistry Group: Prof. Dr. Klaus-Holger Knorr, Henning Teickner
Institute for Geoinformatics: Prof. Dr. Edzer Pebesma
Student research project or thesis opportunity
Students are welcome to participate in this project, either within a research project or a B. Sc. or M. Sc. thesis. Potential topics are:
- Chemical characterization of peat cores (with samples from the archive or within current projects) with elemental analyses and mid infrared spectroscopy and contribution to community data compendia.
- Creation of data compendia from the published literature, including quality assurance.
- Deterministic or probabilistic modeling of peatland processes.
- Literature reviews with the aim to identify knowledge gaps about the effects of temperature, precipitation and N deposition on peatland C accumulation rates.
Requirements
Depending on the specific topic, participants should:
- Have interest in laboratory analyses of peat and the evaluation of the data (topic 1).
- Experiences and interest in programming in R (topics 2 and 3).
- Interest in applying mathematical formulas and software development and (optionally) knowledge in Bayesian statistics (topic 3)
- Interest in learning how to review the scientific literature and identifying research gaps (topic 4).
When
Anytime during the project period (until Spetember 2024).