Next: Acknowledgements
Up: Bayesian Field Theory Nonparametric
Previous: A numerical example
Many nonparametric real world learning problems
are generically non-Gaussian.
Examples include general density estimation
or the reconstruction of forces for quantum systems
from observational data.
Increasing computational resources
allow to solve at least some
of such low-dimensional non-Gaussian
problems directly by discretization.
Figure 1:
(a):
True conditional likelihood
=
.
(b):
Corresponding true field,
here chosen as (conditional) log-likelihood
=
, i.e.,
=
=
.
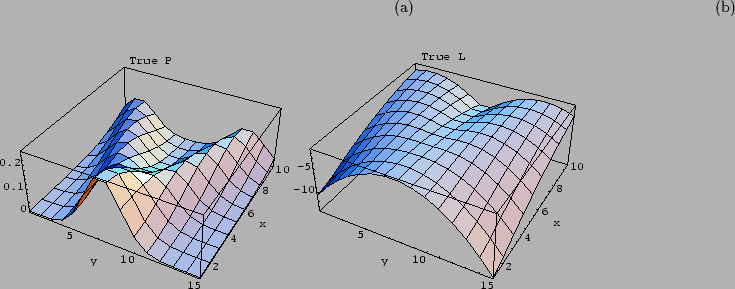 |
Figure 2:
(a):
Conditional empirical density
=
,
as obtained from 50 data points
sampled from the true likelihood
of Fig. 1a under uniform
.
(b): Conditional likelihood
reconstructed from the conditional empirical density
shown on the l.h.s. using, besides the normalization constraint,
an additional Gaussian prior term on
(see text).
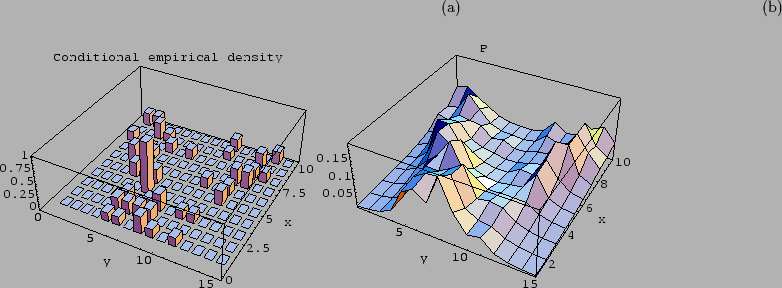 |
Figure 3:
(a): Regression function for
of Fig. 1a.
(b): Regression function for the reconstructed
of Fig. 2b.
(The regression function for a field
is defined as
=
.
The dashed lines indicate the range of
one standard deviation above and below the regression function.)
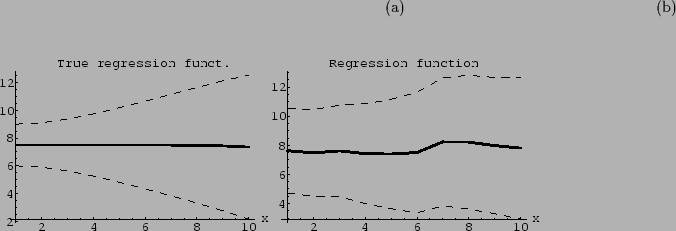 |
Subsections
Next: Acknowledgements
Up: Bayesian Field Theory Nonparametric
Previous: A numerical example
Joerg_Lemm
2000-09-12